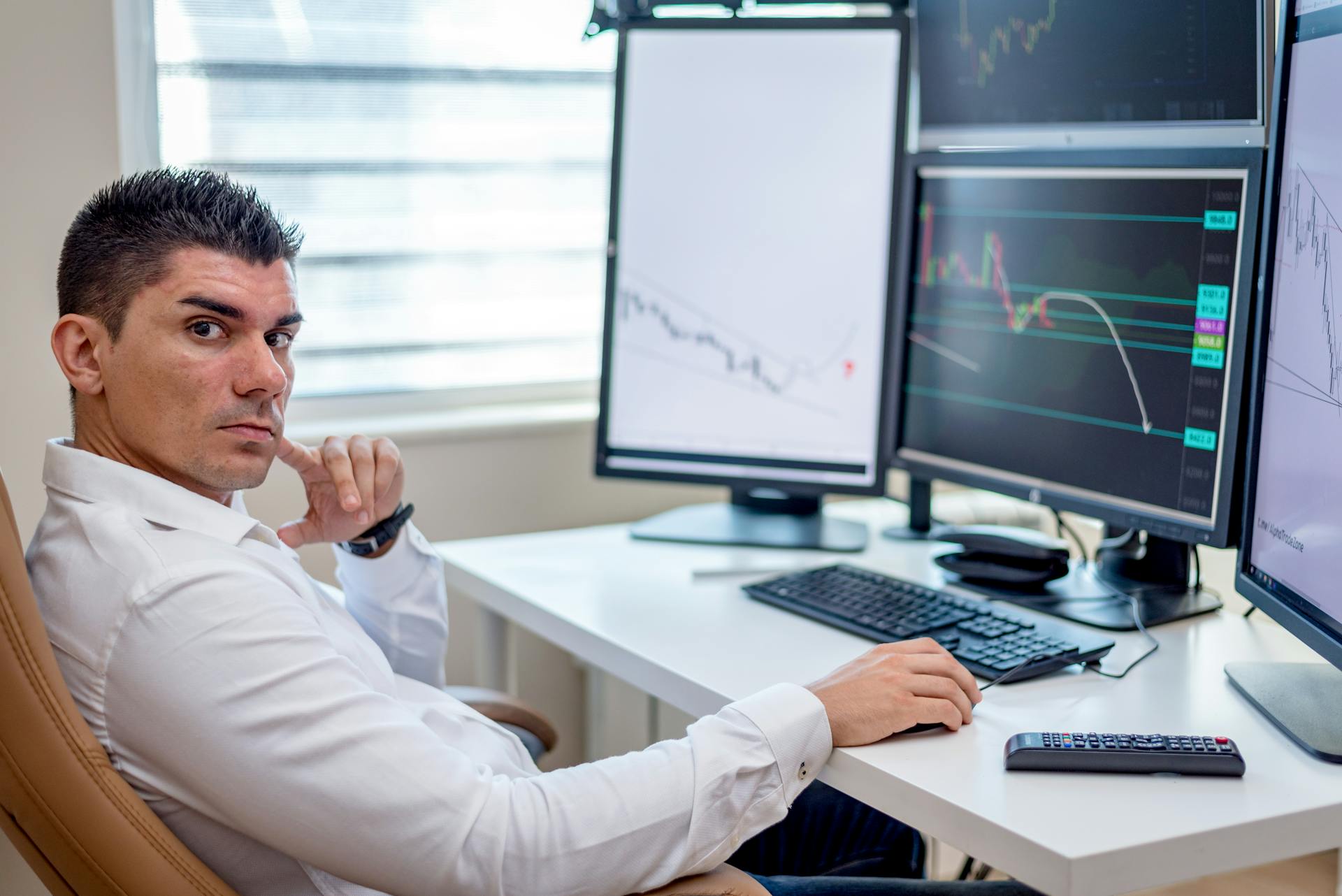
Performing data analysis on datasets is crucial for gaining business insights that can drive informed decision-making. By analyzing data, businesses can uncover hidden patterns and trends that can help them identify areas for improvement and optimize their operations.
According to the article, data analysis can help businesses reduce costs by identifying areas of inefficiency and waste. For example, analyzing sales data can help businesses optimize their pricing strategies and reduce inventory levels.
Businesses that perform data analysis are more likely to stay ahead of the competition. As the article notes, companies that use data-driven insights are 23% more likely to experience revenue growth. This is because data analysis allows businesses to make data-driven decisions that are based on facts rather than intuition.
By gaining a deeper understanding of their customers, businesses can create more effective marketing strategies that drive engagement and conversion. Analyzing customer data can help businesses identify their target audience and tailor their marketing efforts to meet their needs.
A fresh viewpoint: Why Is It Important to Support Local Businesses
Why Data Analysis Matters
Data analysis matters because it helps identify trends and patterns in data, allowing businesses to make informed decisions.
According to a study, companies that use data analysis to inform their decisions are 23% more likely to achieve their goals. This is because data analysis provides a clear understanding of the data, enabling businesses to identify areas for improvement.
Data analysis also helps to reduce costs and increase revenue. For example, analyzing customer data can help businesses identify areas where they can improve customer satisfaction, leading to increased customer loyalty and retention.
A well-analyzed dataset can also help businesses to identify new opportunities for growth. By analyzing market trends and customer behavior, businesses can identify new markets or products to sell.
Data analysis helps to eliminate biases and assumptions in decision-making, leading to more accurate and reliable results. This is especially important in fields like healthcare, where inaccurate assumptions can have serious consequences.
Data analysis can also help to automate decision-making processes, freeing up employees to focus on more strategic tasks.
On a similar theme: New Outlook Make an Email Important
Benefits of Data Analysis
Performing data analysis on datasets is crucial for businesses to make informed decisions and stay ahead of the competition. Accurate data is essential for this process, and having it leads to more reliable outputs from machine learning algorithms.
Having accurate data makes it easier to identify core problems and isolate mistakes without having to wait for high-level signals that something has gone wrong. This saves time and resources that would otherwise be spent on finding and fixing errors.
With accurate data, businesses can gain a competitive advantage by spotting and taking advantage of opportunities faster than their rivals can. This is especially important in a competitive market where businesses need to stay on top of industry trends.
Accurate data also improves customer service by providing a better understanding of customer preferences and interests. This helps businesses prepare for what customers may need assistance with and build a loyal customer base.
You might like: Why Is Accurate Data Collection Important
Here are the benefits of data analysis:
- Improved AI implementation
- Easier identification of core problems
- Competitive advantage
- Improved customer service
- Increased ROI
By having accurate data, businesses can avoid wasting time and money on mistargeted marketing efforts and industry regulations. This saves time, money, and other assets, ultimately leading to increased productivity and efficiency.
Importance of Accuracy
Having accurate data is crucial for any business, and it's essential to perform data analysis on datasets to ensure accuracy. Accurate data helps businesses avoid making decisions based on flawed information.
Inaccurate data can lead to poor decision-making, which can have serious consequences. For instance, using machine learning algorithms with inaccurate data can result in unreliable outputs, making it difficult to trust the results.
Having accurate data allows businesses to identify core problems more easily, isolate and correct mistakes, and stay competitive in the market. It also enables them to provide better customer service by understanding customer preferences and interests.
Here are some benefits of having accurate data:
- Better AI implementation: Using machine learning and AI techniques requires accurate and consistent data to produce reliable outputs.
- Easier identification of core problems: Accurate data helps businesses isolate and correct mistakes without having to wait for high-level signals.
- Competitive advantage: Accurate data helps businesses stay ahead of competitors and industry trends.
- Improved customer service: Accurate data about customer preferences and interests enables businesses to provide better service.
- Increased ROI: Investing in accurate data reduces the need for future investments and lowers costs associated with data.
The Importance of Accuracy
Having accurate data is crucial for businesses to stay competitive and make informed decisions. Inaccurate data can lead to poor decision-making and ultimately harm a company's bottom line.
Modern businesses rely heavily on data, and even small errors can have significant consequences. This is especially true for companies using machine learning and AI techniques to automate processes and build predictive models.
Accurate data is essential for identifying core problems and isolating the root cause of issues. With accurate data, businesses can correct mistakes quickly and avoid wasting time on high-level signals that something has gone wrong.
Having accurate data also provides a competitive advantage, allowing businesses to spot opportunities faster than their rivals. This is especially important in a competitive market where staying ahead of the curve is crucial.
Accurate data is also key to providing excellent customer service. By understanding customer preferences and interests, businesses can tailor their services to meet their needs and build a loyal customer base.
Here are some benefits of having accurate data:
- Better AI implementation
- Easier identification of core problems
- Competitive advantage
- Improved customer service
- Increased ROI
These benefits highlight the importance of accuracy in business operations. By prioritizing data accuracy, businesses can reap numerous rewards and stay ahead of the competition.
Related reading: Why Is Accuracy Important
What Causes Inaccuracy
Data inaccuracy is a natural consequence of a dynamic environment, but certain processes and systems can exacerbate the issue. Things are always changing, so it's impossible to get data 100% right, 100% of the time.
Human error can be a significant contributor to data inaccuracy, especially when manual data entry is involved. Inaccurate data can be caused by things like typos, misunderstandings, or simply not following procedures.
A lack of clear procedures and guidelines can also lead to data quality degradation. Without a clear understanding of what's expected, individuals may not know how to accurately capture data.
Inadequate training can also be a problem, especially if employees are not shown how to use systems and tools correctly. This can result in data being entered incorrectly or not at all.
In some cases, outdated systems or technology can cause data inaccuracy. If systems are not regularly updated or maintained, they can become less effective and more prone to errors.
Related reading: The Most Important Aspect S of a Company's Business Strategy
Data Analysis Techniques
Data analysis techniques are essential for making sense of datasets. Regression analysis is a powerful tool for estimating the relationship between variables.
Regression analysis is used to identify trends and patterns, and it's particularly useful for making predictions and forecasting future trends. It can help you understand whether one variable has an impact on another.
For example, if you work for an ecommerce company, regression analysis can help you determine whether social media spend has a positive correlation with sales revenue. A positive correlation would imply that the more you spend on social media marketing, the more sales revenue you make.
Regression analysis can only determine whether a relationship exists between variables, not cause and effect. So, while a positive correlation may suggest a relationship, it's impossible to draw definitive conclusions based on this analysis alone.
You might like: Important Variable
Techniques
Regression analysis is a powerful technique used to estimate the relationship between a set of variables. It's especially useful for making predictions and forecasting future trends.
Regression analysis can be used to examine the relationship between two variables, such as how much money is spent on social media marketing and sales revenue. This can help inform decisions about the social media budget going forward.
There are many different types of regression analysis, and the model you use depends on the type of data you have for the dependent variable. For example, if your dependent variable is continuous, such as sales revenue in USD, you'd use a different type of regression analysis than if it was categorical in nature, such as customer location by continent.
Cohort analysis is another technique that groups users based on a shared characteristic, such as the date they signed up for a service or the product they purchased. This allows analysts to track their behavior over time and identify trends and patterns.
By examining customer behavior in the context of the customer lifecycle, cohort analysis can help companies tailor their service to specific customer segments. This can lead to more targeted and personalized marketing efforts.
Regression analysis can only be used to determine whether or not there is a relationship between a set of variables – it doesn't tell you anything about cause and effect. So, while a positive correlation between social media spend and sales revenue may suggest that one impacts the other, it's impossible to draw definitive conclusions based on this analysis alone.
Cohort analysis is dynamic, allowing you to uncover valuable insights about the customer lifecycle. This can help companies optimize their service offerings and marketing to provide a more targeted experience.
Explore further: Why Are Surveys Important for Companies
Quantitative vs Qualitative
Quantitative data is anything measurable, comprising specific quantities and numbers.
Quantitative data includes sales figures, email click-through rates, number of website visitors, and percentage revenue increase.
This type of data is often used to explain certain phenomena or make predictions. Quantitative analysis techniques focus on the statistical, mathematical, or numerical analysis of large datasets.
Qualitative data, on the other hand, cannot be measured objectively and is open to more subjective interpretation.
Qualitative data includes comments left in response to a survey question, things people have said during interviews, tweets and other social media posts, and the text included in product reviews.
Data analysts work with both quantitative and qualitative data, so it’s essential to be familiar with a variety of analysis methods.
For more insights, see: Why Is Quantitative Data Important
Time Series
Time Series is a statistical technique used to identify trends and cycles over time, helping analysts forecast future fluctuations in a variable of interest.
Time series data is a sequence of data points measuring the same variable at different points in time, such as weekly sales figures or monthly email sign-ups.
Explore further: Why Are Choke Points Important
Trends are stable, linear increases or decreases over an extended time period, while seasonality refers to predictable fluctuations due to seasonal factors over a short period of time.
Seasonal patterns can be seen in data such as a peak in swimwear sales in summer around the same time every year.
Cyclic patterns, on the other hand, are unpredictable cycles where the data fluctuates due to economic or industry-related conditions.
Time series analysis has immense value for business, and is used across various industries for stock market analysis, economic forecasting, and sales forecasting.
These models are typically classified into three broad types: autoregressive (AR) models, integrated (I) models, and moving average (MA) models.
Here are the main patterns to look out for in time series data:
- Trends: Stable, linear increases or decreases over an extended time period.
- Seasonality: Predictable fluctuations in the data due to seasonal factors over a short period of time.
- Cyclic patterns: Unpredictable cycles where the data fluctuates due to economic or industry-related conditions.
Sentiment
Sentiment analysis is a powerful tool that can help you understand how your customers feel about your brand, product, or service. It's a type of text analysis that involves interpreting and classifying the emotions conveyed in textual data.
Many companies overlook the value of qualitative data, but sentiment analysis can reveal untold insights. This technique is highly useful for businesses that want to understand customer opinions and emotions.
There are three main types of sentiment analysis models: fine-grained sentiment analysis, aspect-based sentiment analysis, and presumably others. Fine-grained sentiment analysis allows you to focus on opinion polarity in depth, categorizing ratings along a scale from very positive to very negative.
For example, if you're analyzing customer reviews, fine-grained sentiment analysis can help you understand the nuances of their opinions. Aspect-based sentiment analysis, on the other hand, identifies the specific aspects of your product or service that customers are referring to, such as a certain feature or ad campaign.
Sentiment analysis uses Natural Language Processing (NLP) algorithms and systems to associate certain inputs with certain outputs. For instance, the word "annoying" would be recognized and tagged as "negative".
Suggestion: What Is the Most Important Aspect of a Website
Cleaning the Data
Cleaning the data is a crucial step in the data analysis process. It's a phase that will take up the most time, and it's essential to get it right to ensure accurate results.
On a similar theme: When Communicating It's Important to
You'll likely be removing major errors, duplicates, and outliers from your data. This can be a tedious task, but it's necessary to maintain the integrity of your data.
Removing unwanted data points is also a significant part of the cleaning process. This can include irrelevant or redundant data that doesn't add value to your analysis.
Structuring the data is another important aspect of cleaning. This involves fixing typos, layout issues, and other formatting problems that can affect the accuracy of your analysis.
Filling in major gaps in data is a critical task that requires attention to detail. You'll need to carefully examine your data to identify missing information and make informed decisions about how to handle it.
Here are some common tasks you'll encounter during the data cleaning process:
- Removing major errors
- Removing duplicates and outliers
- Removing unwanted data points
- Structuring the data
- Filling in major gaps in data
Visualizing and Sharing Findings
Visualizing and sharing your findings is a crucial step in data analysis. This is where you take the insights you've gained and present them in a way that's easy for others to understand.
Data visualization is the key to making complex data more understandable. It involves presenting data in charts and graphs instead of tables or databases.
You can use a data visualization tool like Tableau or Microsoft Power BI to create interactive and dynamic visuals. These tools make it easy to turn millions of data points into simple visuals that guide decision-making.
Standard Excel graphs and charts are also a great option for data visualization. You can use them to create bar charts, line graphs, and more.
Interactive Excel graphs are another way to visualize data. They allow viewers to explore the data in more detail and gain a deeper understanding of the insights.
There are various ways to visualize data, including using a tool like D3.js built using JavaScript. This is a great option for creating web-based visualizations.
Data visualization can be a game-changer for businesses and organizations. For example, a marketing agency used data visualization to track the performance of client campaigns, retaining clients and attracting new ones.
A data visualization tool like Google Charts or Tableau can help you share your findings with others. These tools make it easy to create visualizations that are easy to understand and share.
Here are some of the most common types of data visualization:
- Bar charts
- Line graphs
- Scatter plots
- Heat maps
Data Analysis Process
Business data analytics collects, processes, and analyzes data to help make smart decisions.
By looking at past data, businesses can predict what's coming next, helping them act before problems pop up.
Using sales forecasting techniques, a retail store might check past sales to determine which products will be hot sellers during the holidays.
Automation and cloud computing make it easier to streamline business processes and cut costs.
A manufacturing company might dig into production data to spot bottlenecks, making things run cheaper through workflow automation and supply chain optimization.
Business intelligence tools often aid the predictive process by analyzing data to identify patterns and trends.
Customer segmentation and sentiment analysis algorithms help companies understand what customers like, so they can tailor their offerings.
Data analytics sparks creativity and product development by spotting new trends in what consumers want.
A tech startup might use deep learning models, real-time analytics, and statistical analysis to find gaps in the market, leading to a new app nobody saw coming.
Take a look at this: Why Automation Is Important
Analyzing data helps businesses make informed decisions, predict future outcomes, and identify areas for improvement.
By automating processes and streamlining operations, businesses can cut costs and increase efficiency.
Companies that use data analytics to personalize customer experiences often see increased customer loyalty and retention.
Data analysis can help businesses predict sales trends, identify new markets, and develop innovative products and services.
Check this out: Why Is a Target Market Important to Businesses and Organizations
Tools for Data Analysis
To perform data analysis on datasets, you need the right tools for the job. Every phase of the data analysis process requires a variety of tools to gain valuable insights from data.
A data analyst's toolkit is not complete without Microsoft Excel, which is a powerful tool for data analysis. Python is another essential tool, especially for data scientists who want to work with complex data sets.
R is a popular programming language used for statistical computing and data visualization. Jupyter Notebook is a great tool for data analysts to work with Python, R, and other languages.
For your interest: Why Workplace Ethics Is Important
Apache Spark is an open-source engine for large-scale data processing, while SAS is a comprehensive data analysis software. Microsoft Power BI and Tableau are powerful tools for data visualization and business intelligence. KNIME is another tool that helps data analysts to work with complex data sets.
The top tools for data analysts include:
- Microsoft Excel
- Python
- R
- Jupyter Notebook
- Apache Spark
- SAS
- Microsoft Power BI
- Tableau
- KNIME
Data Analysis in Specific Industries
Data analysis is a crucial aspect of various industries, where it helps identify trends and patterns that can inform business decisions. In the healthcare industry, data analysis can help medical professionals identify high-risk patients and provide targeted interventions.
For instance, a study on patient outcomes showed that data analysis helped reduce hospital readmissions by 20%. In the finance sector, data analysis can help identify potential risks and opportunities, such as predicting stock market fluctuations.
In the manufacturing industry, data analysis can help optimize production processes, resulting in increased efficiency and reduced costs. Companies like Amazon use data analysis to optimize their supply chain and delivery processes, resulting in faster and more reliable shipping.
Expand your knowledge: What Is an Important Factor That Help Determines Cost
Develops and Preserves Brand Credibility
Accurate data is essential for building trust with both internal and external stakeholders. Internally, quality data helps make a company more productive, reliable, and successful.
Quality data properly managed helps show customers that your organization is responsive to their needs. It also indicates that your company takes their security seriously and provides reliable information.
Accurate data simplifies compliance with ever-changing industry regulations. This is crucial for maintaining a good reputation and avoiding costly fines.
On a similar theme: Why Are Values Important in a Company
Big in Healthcare Considerations
Modern analytics offers the possibility of not only analyzing historical data, but also predicting future outcomes with evidence-based actions.
Everyone in the healthcare industry is looking for ways to do more with fewer resources, which is driving the pursuit of data analysis to reduce risk, detect fraud, and improve efficiency.
Payers and suppliers are using data analysis to save lives, making it a critical consideration in the industry.
The emphasis on reform has prompted healthcare stakeholders to focus on data analysis, which can yield the greatest results in areas such as reducing risk and detecting fraud.
By leveraging enhanced data and analytics, healthcare stakeholders can improve efficiency, save lives, and make the most of limited resources.
For more insights, see: Why Is Stakeholder Important
Insurance Profitability Tool
We built a custom data analysis tool for a U.S. insurance provider that helped them make sense of large amounts of data. Our tool uses real-time data processing and easy-to-use dashboards for flexible filtering.
The tool provides insights into insurance profits and losses across different industries, which was a major challenge for the insurance provider. Our team developed a predictive model that uses historical data to identify insurance cases.
The reporting system highlights key factors and profitability based on various metrics, giving the insurance provider valuable insights for their business. The tool also consolidates data from multiple sources, making it easier for the provider to analyze their data.
The insurance provider was impressed with the solution, praising the team for providing an excellent solution for creating valuable insights for their business.
On a similar theme: Why Is Travel Insurance Important
Frequently Asked Questions
What is the main purpose of data analysis?
Data analysis helps make informed decisions by extracting useful information from data. It empowers decision-making with accurate insights.
What is the most important key benefit of data analysis?
The most important key benefit of data analysis is identifying potential issues and optimizing processes to increase revenues and achieve better outcomes. By leveraging data insights, businesses can make informed decisions and stay ahead of challenges.
Sources
- https://dataforest.ai/blog/the-importance-of-data-analytics-in-todays-business-world
- https://pmc.ncbi.nlm.nih.gov/articles/PMC8733917/
- https://www.safegraph.com/guides/why-accurate-data-is-important
- https://careerfoundry.com/en/blog/data-analytics/data-analysis-techniques/
- https://www.makeuseof.com/tag/what-is-data-analysis/
Featured Images: pexels.com