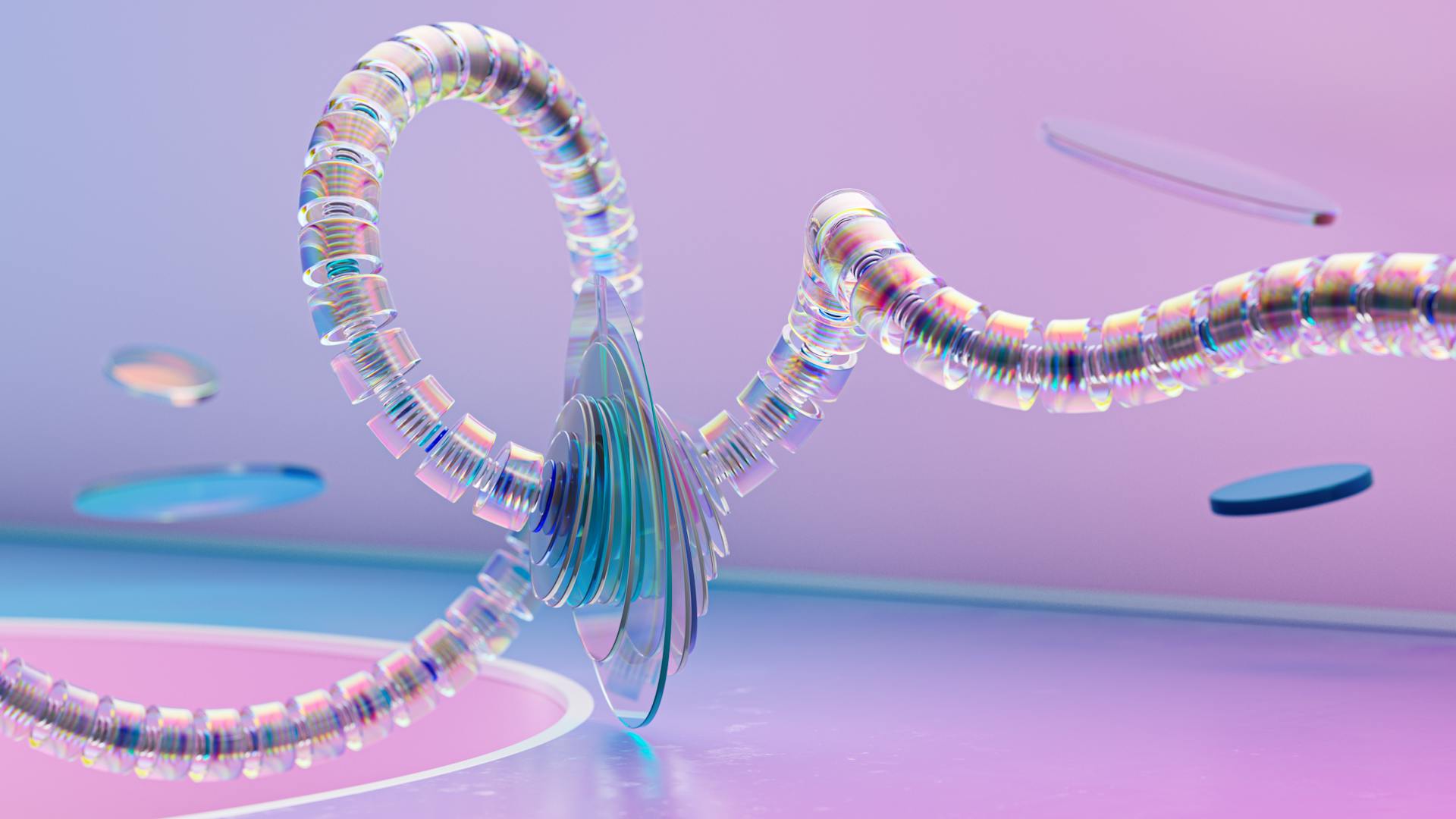
Data validation is more than just a necessary step in the data management process - it's a crucial one. Without it, inaccuracies and errors can spread quickly, causing problems downstream.
Inaccurate data can lead to incorrect conclusions, wasted resources, and even financial losses. For example, a study found that a single typo in a database entry can result in a 100% increase in errors.
Data validation helps ensure that the information we collect is accurate and reliable. This is especially important in industries where data is used to make critical decisions, such as healthcare or finance.
By verifying data at every step, we can prevent errors from occurring in the first place.
You might enjoy: Why Validation Is Important
Why Data Validation is Important
Data validation is a crucial process that ensures the accuracy, completeness, and consistency of data. It helps eliminate errors and inaccuracies, which can lead to costly mistakes and wasted resources.
Inaccurate and incomplete data can erode employee confidence and make organizations reluctant to use their data. In fact, companies waste $180,000 annually on undeliverable mail because four percent of their mailing-list addresses are inaccurate. This highlights the importance of having reliable data.
Data validation can reduce the time spent cleaning bad data later on, allowing analysts and engineers to focus on more valuable tasks. It also helps organizations avoid making decisions based on inaccurate or incomplete data, which can lead to costly mistakes.
Data validation is a team effort that requires collaboration among everyone involved in the data life cycle. It can be done at multiple points in the data life cycle, and it's essential to confirm that the data is correct to ensure the highest quality data is being produced.
By implementing data validation, organizations can save time and money, improve employee confidence, and make informed decisions based on reliable data. This is especially important in today's competitive market, where accurate data insights are crucial for success.
Here are some key benefits of data validation:
- Reduces the time spent cleaning bad data
- Helps organizations avoid making decisions based on inaccurate or incomplete data
- Improves employee confidence
- Saves time and money
- Ensures the highest quality data is being produced
Data Validation Process
The data validation process is a crucial step in ensuring the accuracy and integrity of data within a system. It's a four-step process that helps prevent data entry errors and improves user experience.
The four steps of the data validation process are: data entry, validation rules definition, validation process, and error handling. These steps work together to provide immediate feedback to users as they enter data, helping them correct errors as they occur.
Data entry is the first step, where users input data into the system. Validation rules definition comes next, where specific rules are established to ensure data accuracy. The validation process then checks the entered data against these rules, identifying any errors or inconsistencies. Finally, error handling takes place, where the system provides feedback to users and guides them through the correction process.
The system enforces validation rules in real-time, providing immediate feedback to users as they enter data. This helps prevent data entry errors and improves data accuracy.
Here's a breakdown of the four-step data validation process:
- Data entry: Users input data into the system.
- Validation rules definition: Specific rules are established to ensure data accuracy.
- Validation process: The system checks the entered data against these rules.
- Error handling: The system provides feedback to users and guides them through the correction process.
The 9 Benefits
Data validation is a critical process that ensures the integrity of your data, safeguarding it from errors, inconsistencies, and inaccuracies. By applying validation rules, you can ensure that the data entered or imported meets specific quality standards, reducing inaccuracies, inconsistencies, and errors in the data.
Improved data quality is one of the key benefits of data validation. According to Example 4, data validation is crucial for maintaining the accuracy and reliability of data within a system. By ensuring that the data meets specific quality standards, you can reduce inaccuracies, inconsistencies, and errors in the data, resulting in a higher overall data quality.
Data validation also prevents errors from occurring in the first place. By enforcing consistency in data elements, validation rules ensure that data is required to conform to predefined standards, such as data types, formats, and ranges. This is essential for reporting, analysis, and data integration, as it ensures that data elements are uniform and can be reliably compared and combined.
Here are the 9 benefits of data validation:
By implementing data validation, you can ensure that your data is accurate, complete, and reliable, leading to improved decision-making, reduced errors, and increased productivity.
Challenges and Approaches
Data validation can be a time-consuming process, especially when done manually. Validation by Scripting, for instance, requires writing an entire script in a programming language like Bash or Python, which can be a tedious task.
However, data validation is crucial for ensuring accuracy and quality of data. It also ensures that the data collected from different resources meet business requirements.
Some data validation techniques include Validation by Scripting, Validation by Grafana, and Data Validation in Excel. These methods can be used to perform data validation tasks, but they have their own set of challenges.
Here are some of the popular data validation tools, along with their characteristics:
- Validation by Scripting: A time-consuming process that requires verification
- Validation by Grafana: A comparison dashboard that can be created to fetch data from a desired database
- Data Validation in Excel: A manual task that involves applying formulas on cells
Challenges
Validating data can be a time-consuming task, especially when dealing with large databases. In fact, sampling the data for validation can help to reduce the time needed.
Data validation can be challenging because data may be distributed in multiple databases across a project. This can make it difficult to ensure that all data is accurate and complete.
As companies adopt a data-driven approach, data accuracy and completeness become increasingly important. Data validation becomes a huge task when the number of columns in datasets increases.
Here are some common challenges that data validation can pose:
- Validating data format can be extremely time-consuming.
- Data may be distributed in multiple databases across a project.
- Data validation becomes a huge task when the number of columns in datasets increases.
In some cases, data validation may require validating data from multiple sources simultaneously. This can help prevent conflicts and errors between datasets.
Approaches and Techniques
Data validation is a crucial step in ensuring the accuracy and quality of data. It's essential to use the right techniques to perform data validation effectively.
One popular approach is validation by scripting, where the validation process is performed through a scripting language like Bash or Python. This method requires writing an entire script for the validation process, which can be time-consuming and requires verification.
Data validation can also be done using Grafana, a tool that allows you to create a comparison dashboard to fetch data from a desired database and display it in the form of a table or graph.
Another manual approach is using data validation in Excel, where you can apply required formulas on cells to perform data validation tasks. This method is widely accepted in many organizations, but it can be a time-consuming process.
Here are some common data validation tools:
- Validation by Scripting: uses scripting languages like Bash or Python
- Validation by Grafana: creates a comparison dashboard to fetch data from a database
- Data Validation in Excel: applies required formulas on cells
Fighting Decay
Good data is hard to come by, especially as time goes on. Data decay is a natural process where data that was once accurate becomes outdated.
Data decay happens over time due to changes in user information, such as a user's address changing, or a business starting to collect new data fields that are incomplete for existing users.
Validating data can help reduce errors caused by data decay. It identifies missing, incomplete, inconsistent, and inaccurate data.
Data validation at the client or processing state won't help with decay because data changes over time and should be constantly updated in the warehouse.
By validating data, you can regain the trust that might be lost in your organization and create a better customer experience by targeting advertisements, emails, and calls to customers based on their potential needs.
Here are some common causes of data decay:
- User information changes
- New data fields are added that are incomplete for existing users
- Data is not updated in the warehouse
By being proactive and validating data, you can prevent data decay and ensure that your data remains accurate and reliable.
Featured Images: pexels.com